Artificial intelligence (AI) has rapidly become a highly disruptive tool in several industries and scientific research fields, providing scientists and engineers with extremely powerful capabilities only dreamed of a mere couple of decades ago. This article will examine how AI transforms ore analysis and enables precision mining.
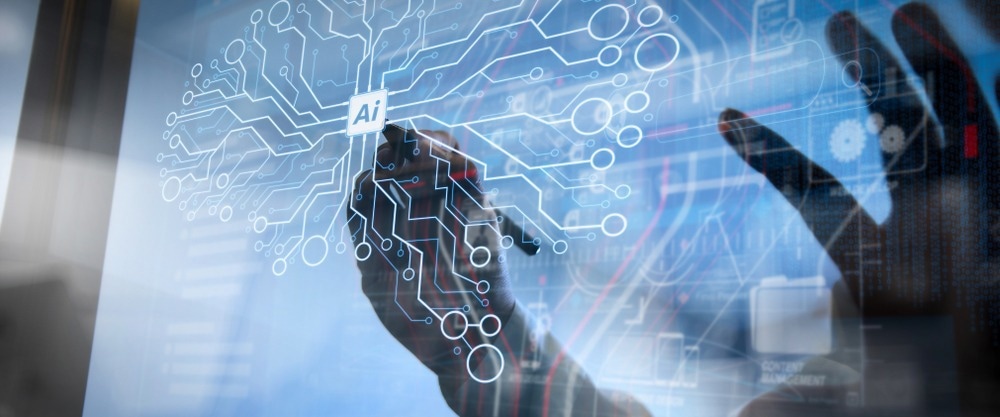
Image Credit: everything possible/Shutterstock.com
Why is Ore Analysis Important?
Mines operate within extremely tight financial margins, where multiple factors affect the profitability of operations. Vast amounts of ore are extracted daily, either from deep underground or surface deposits, and these typically contain only a minuscule amount of financially viable resources.
Ore samples are extremely heterogeneous, with the bulk of the material comprising largely useless components such as soil, unusable rock, and various contaminants. Extracting viable minerals from these samples is a lengthy, costly, and complex process.
Various types of physical, chemical, mechanical, and laboratory-based sorting, preparation, and analytical methods have been developed over the decades to analyze the composition of bulk and prepared ore samples. Each method has its distinct advantages and drawbacks, involving varying degrees of time and cost, and some are more accurate than others.
Techniques commonly employed in ore analysis include optical image analysis, optical microscope point counting, X-Ray diffraction, X-Ray fluorescence spectroscopy, scanning electron microscopy, and electron probe microanalysis. While these tools have proven extremely successful, the industry is now looking toward the future and the advent of precision mining.
The Role of AI in Mining
AI encompasses a range of related technologies, including algorithms, neural networks, the Internet of Things, deep learning, and machine learning. These technologies have been extremely disruptive in numerous industries, improving the accuracy and speed of analysis and offering significant cost, efficiency, and performance benefits.
Advanced AI algorithms excel in analyzing vast data sets compared to human operators, enhancing resource prediction, thereby improving planning and increasing the return on investment for mining companies.
Machine learning, deep learning, and algorithms also enable the development of superior models, helping companies gain unique insights that are not possible with conventional technologies, ultimately aiding profitability. AI models can be trained on vast amounts of previous data, enhancing image recognition and other vital elements of the analytical process.
AI-augmented vision technologies are already being employed in the mining industry to automatically identify the types of minerals present in rock samples with great precision. This process is considerably faster than manual analysis and label sampling.
The utilization of AI tools in the analysis of ore samples significantly improves the quality of the process and the quantity of material that can be processed at the same time. Mineral composition can be determined with a much higher degree of accuracy, speed, and at lower cost than previously possible, thereby enhancing the profitability of mining projects.
Applying AI to Improve the Prediction of Copper Recovery
A paper published in 2020 explored the use of AI to improve copper recovery prediction. As with all sectors in the industry, copper mining is undergoing a revolution in the age of Industry 4.0.
Leaching is a commonly used method for copper recovery, but this process is facing new regulations in nations like Chile aimed at improving the recovery rate of critical resources.
Using data from northern Chile, the researchers developed two models—one based on real-world data and the other on piling. AI techniques, such as random forest, were employed to improve the prediction of copper recovery rates using leaching. The results of this study demonstrate a 98.90 % value for operational data and 98.72 % for piling data.
The authors stated that the AI-based methodology utilized could be transferred to other piling columns with varying characteristics and leaching processes for different types of minerals. While some adjustments would be necessary, the methodology has the potential to be employed in different mines.
Deep Learning Used for the Intelligent Identification and Prediction of Mineral Resources in Deposits
Training deep learning algorithm networks and image processing technologies to accurately analyze and sort images of critical mineral deposits poses numerous challenges.
For instance, there is a scarcity of image samples with mineral point labels suitable for training, and various features are irregular and small, with images being highly similar.
To address these issues, a team published a paper in Sustainability in June 2023. Their research resulted in a multiscale feature attention framework (MFAF), which utilizes deep learning methods to improve image analysis and recognition for precise ore analysis.
The paper demonstrated that this new deep learning method could, to a degree, overcome the central challenge of a lack of useful mine label images for training.
Numerous experimental results also demonstrated that MFAF has several advantages over other innovative AI-based methods for mineral sample image recognition. However, the authors recognized limitations associated with the research conditions.
Future Outlooks of AI in Mining
The use of AI-based technologies, including deep learning, machine learning, and neural networks, for various ore analysis methods is still in its relative infancy. However, recent research has demonstrated the potential improvements in efficiency, accuracy, and cost benefits AI can bring to the mining industry.
References and Further Reading
Gao, L., et al. (2023). Intelligent Identification and Prediction Mineral Resources Deposit Based on Deep Learning. Sustainability. doi.org/10.3390/su151310269.
Vining, K. (2017). Understanding Iron Ore Analytical Tools. [Online] CSIRO. Available at: https://research.csiro.au/resourcesandsustainability/iron-ore-analytical-tools/.
Flores, V., Keith, B., Leiva, C. (2020). Using Artificial Intelligence Techniques to Improve the Prediction of Copper Recovery by Leaching. Journal of Sensors. doi.org/10.1155/2020/2454875.
Disclaimer: The views expressed here are those of the author expressed in their private capacity and do not necessarily represent the views of AZoM.com Limited T/A AZoNetwork the owner and operator of this website. This disclaimer forms part of the Terms and conditions of use of this website.