The mining industry is embracing advanced technologies like hyperspectral imaging and artificial intelligence for innovative mineralogical mapping of ores. This transformation enhances our capability to analyze and characterize ore bodies, contributing to improved efficiency and sustainability in mineral exploration and processing.
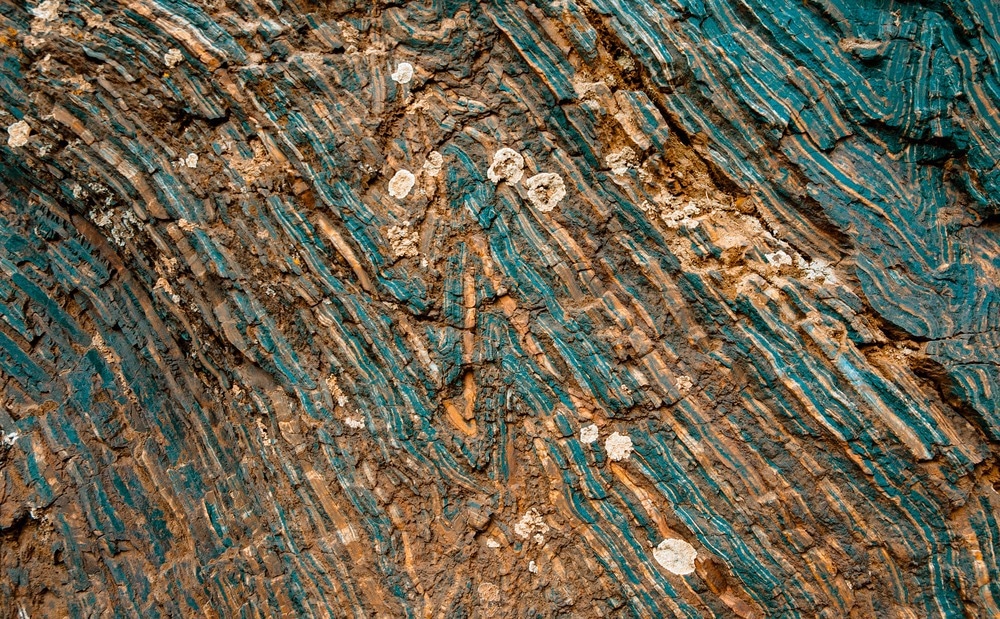
Image Credit: Repina Valeriya/Shutterstock.com
The Transformation of Mineralogical Mapping
Traditionally, analyzing ore bodies' texture, composition, and structure relied heavily on visual inspection of drill core samples and microscopic analysis. While providing useful information, these techniques were highly localized, slow, labor-intensive, and delivered data sets prone to uncertainty and human error.
For example, traditional lab assays often lead to inadequate process designs, as they fail to capture the inherent variability within ore deposits and lack complete quantified mineralogical information essential for metallurgical modeling.
Recent innovations in mineralogical mapping through hyperspectral imaging, scanning electron microscopy, and spectrometry are overcoming these long-standing challenges. Now, entire drill cores and sample sets can be scanned to produce high-resolution 3D imaging and quantitative mineralogical data mapped across complete ore bodies.
Hyperspectral Imaging Revolutionizes Ore Analysis
Hyperspectral sensors measure reflected solar radiance in hundreds of narrow spectral bands, allowing the construction of an essentially continuous reflectance spectrum for each image pixel. This enables detailed chemical and mineral characterization by matching spectral signatures to known mineral spectra.
The latest generation of satellite hyperspectral sensors, such as the Italian Space Agency's Prisma, can scan large exploration areas from space at higher resolutions. These systems allow geologists to map mineral alterations, lithologies, and associated minerals cost-effectively over vast greenfield terrains during early exploration to pinpoint drilling targets.
Once promising ore bodies are identified, close-range hyperspectral core and face imaging generate crucial data on mineral variability within an ore zone.
Artificial Intelligence Reveals Subtle Relationships
While hyperspectral data provides vital mineralogical information, realizing its full potential requires sophisticated analysis to uncover subtle patterns in integrated geological, chemical, and mineral datasets. Modern artificial intelligence approaches are proving invaluable for this task.
Self-organizing maps (SOMs) help cluster, classify, and visualize high-dimensional ore characterization data to reveal key relationships between gold occurrence, grade, and attributes like grain associations and exposure. By preserving topological relationships, SOMs allow geologists to interpret complex statistical interconnectivities.
Integrating hyperspectral mineral data with chemical and metallurgical assays in SOM analysis enhances understanding of an ore body's genesis and downstream processing behavior.
Micro LIBS and XRF in Ore Characterization
In the last two decades, advancements in mineralogical mapping for ore analysis have been driven by innovative techniques like micro X-Ray fluorescence (μXRF) and micro Laser-Induced Breakdown Spectroscopy (μLIBS).
These spectroscopic methods achieve accurate imaging with ppm-level sensitivity at speeds surpassing conventional techniques, making them particularly suitable for complex mineralogizes. In addition, the commercialization of benchtop systems and user-friendly software has increased their accessibility, making them suitable for large samples with high resolution.
Recent progress in portable hyperspectral devices, integrated with handheld XRF analyzers, enables rapid in-situ ore analysis. These systems, equipped with near-infrared (NIR) or short-wave infrared (SWIR) spectrometers, function as stand-alone geological tools, enhancing field mineralogical mapping grade control and eliminating delays in sample transportation and offsite analysis.
Recent Research and Development
Efficient hyperspectral mineralogical mapping using clustering-matching and NMF
A study published in Remote Sensing introduces a novel approach, unsupervised clustering-matching mapping (KSM), combining k-means and spectral matching (SM) techniques for hyperspectral mineral mapping. The method addresses limitations in SM, such as poor accuracy due to noise and inefficient per-pixel matching.
The proposed KSM incorporates nonnegative matrix factorization (NMF) for feature extraction, enhancing mapping efficiency.
The experimental results, using an airborne hyperspectral image of Cuprite, Nevada, demonstrate that KSM significantly outperforms SM in terms of mapping accuracy, achieving approximately 35% higher accuracy on average. This enhanced precision is crucial for identifying and characterizing minerals within ore deposits.
The substantial reduction in mapping time, exceeding 95% compared to SM, enhances the efficiency of ore analysis, enabling quicker decision-making in mining and mineral exploration.
KSM's independence from mixing different mineral types makes it versatile and applicable to various ore compositions, ensuring its adaptability across diverse deposits. In addition, while maintaining mapping detail, the method's noise reduction capabilities are essential for distinguishing valuable minerals from background noise in ore analysis.
Overall, the proposed method significantly enhances the efficiency, accuracy, and adaptability of mineralogical mapping, offering valuable improvements for ore analysis in mining and exploration.
Precision analysis of gold ores with AI
A study published in Mining explores the application of self-organizing maps (SOM), a type of artificial neural network (ANN), in analyzing and correlating data from process mineralogical studies of gold ore.
Using SOM, researchers identified strong positive correlations among variables such as accessibility, median gold grain diameter (D50), exposed perimeter, and arsenic contents. This highlights SOM's ability to discern significant large-scale spatial chemical–mineralogical patterns in gold ore, contributing to identifying vital indicator variables for ore processing in geometallurgy.
The study represents a significant step in data integration, enhancing the interpretation and understanding of key variables in developing a predictive geometallurgical model for ore processing.
Challenges and Future Outlooks
The progress in ore analysis is notable, yet significant areas for improvement exist.
Reconciling multi-scale data integrating modal mineralogy from various sources remains a challenge, requiring collaborative data science efforts. Techniques for analyzing trace minerals below detection limits are needed to understand their processing impact, requiring advancements in detection thresholds. Lastly, integrating mineral mapping with early-stage mineral processing principles is crucial for geometallurgy advancements.
Addressing these gaps could lead to transformative technologies like real-time sensing for advanced process control and AI-driven adjustments, revolutionizing the processing of challenging assets.
References and Further Reading
Ren, Z., Zhai, Q., & Sun, L. (2022). A novel method for hyperspectral mineral mapping based on clustering-matching and nonnegative matrix factorization. Remote Sensing, 14(4), 1042. https://doi.org/10.3390/rs14041042
Costa, F. R., Carneiro, C. D. C., & Ulsen, C. (2023). Self-Organizing Maps Analysis of Chemical–Mineralogical Gold Ore Characterization in Support of Geometallurgy. Mining, 3(2), 230-240. https://doi.org/10.3390/mining3020014
Fabre, C., Trebus, K., Tarantola, A., Cauzid, J., Motto-Ros, V., & Voudouris, P. (2022). Advances on microLIBS and microXRF mineralogical and elemental quantitative imaging. Spectrochimica Acta Part B: Atomic Spectroscopy, 194, 106470. https://doi.org/10.1016/j.sab.2022.106470
Rani, N., Kumar Ahirwar, S., Anoop, V. M., & Krishnamurthy, K. V. (2023). Mineral Mapping through Advanced Airborne Hyperspectral Remote Sensing Techniques. https://doi.org/10.5772/intechopen.111831
Disclaimer: The views expressed here are those of the author expressed in their private capacity and do not necessarily represent the views of AZoM.com Limited T/A AZoNetwork the owner and operator of this website. This disclaimer forms part of the Terms and conditions of use of this website.