Every day, mining operations confront challenges that reduce productivity, safety, and sustainability. These challenges include geological concerns, unsafe work environments, and substantial operational costs. As the demand for minerals and metals continues to rise, these restrictions increase the pressure for innovative solutions.
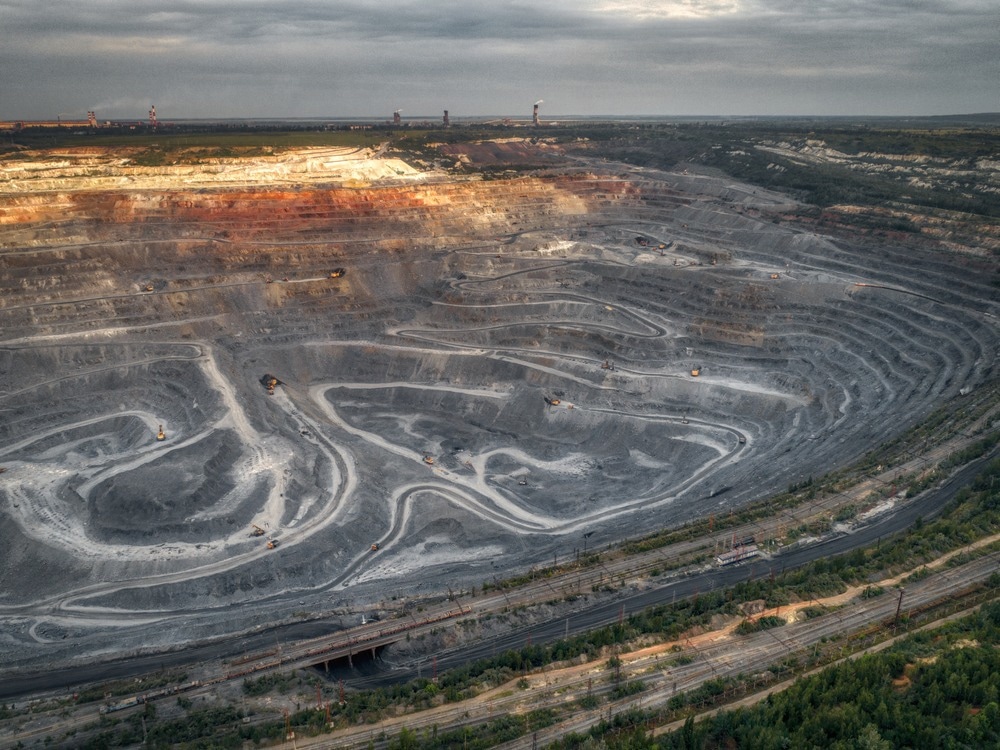
Image Credit: Nordroden/Shutterstock.com
Enter artificial intelligence (AI). As witnessed in numerous other industries, AI and machine learning offer growing potential for optimizing mining operations in various ways.
For example, mining companies can use machine learning for enhanced ore analysis and thus improved extraction operations. The technology can also be employed to reap valuable insights from satellite and drone images, which can be then used to improve operational robotics.
Improving Resource Extraction in Mining with AI-Powered Ore Analysis
Ore grade estimation involves projecting the quantity of minerals that could be extracted from a geologic deposit.
Traditional approaches to this process involved taking manual samples and performing assays, which can be labor-intensive and have a wide margin of error. A more recent approach involves the creation of complex geostatistical models that provide greater precision.
These models demand specialized expertise to process large amounts of complex data. There is also significant variability and uncertainty associated with geostatistical modes.
Machine learning offers a superior approach to ore grade estimation through feature extraction. This involves combining various datasets to provide highly accurate predictions.
By focusing on certain factors, such as geological features and the presence of particular minerals, machine learning algorithms continuously improve the predictions they generate. The accuracy of these predictions improves with the increasing amount of data processed by the machine learning system. These predictions can then be used to inform resource extraction operations.
Machine learning and mining also improve resource extraction by analyzing trace elements obtained through laser ablation–inductively coupled plasma–mass spectrometry (LA–ICP–MS).
The analysis of these trace elements can reveal the origins of ore deposits. This information can then be used to improve resource extraction. Researchers used machine learning and LA–ICP–MS in one study to analyze pyrite trace element data. Results demonstrated that this approach was a valid predictor of ore deposit type.
Another approach to improving resource extraction through machine learning involves the identification of mineral ore based on hyperspectral images of mine excavation faces. In one study, researchers used machine learning to analyze two types of hyperspectral images: one set acquired in a laboratory setup with optimal conditions, and one set stimulating a mine face in a simulated field setup.
These machine-learning models were able to classify laboratory spectra with an overall accuracy rate of 98 %. The accuracy in the simulated setup was lower than that in the laboratory setup; however, the researchers attributed this to the likely impact of lower spatial resolution in the simulated field setup.
The study confirmed the validity of employing this approach to document the spatial distribution of ore minerals in an excavated mine face.
Better Extraction Through AI-Powered Geospatial Analysis and Robotics
Machine learning can also improve resource extraction operations through geospatial analysis. By evaluating images from satellites and drones, machine-learning systems can identify mineral-rich zones. In so doing, these geospatial systems can accelerate the exploration process and reduce the risk of drilling in areas with unknown geological qualities.
In a recent study published in Ore Geology Reviews, researchers used Geographic Information System-based machine learning to create accurate maps of prospective mineral deposits. The team achieved these results by creating a model of copper deposits and information on spatial proxies related to ore-forming processes.
These results demonstrate that the team successfully created models with high predictive precision. The study team also produced numerous targets for exploration in different provinces in China. The researchers concluded that this approach could be applied to other mineral systems and regions.
Robotics in the mining industry is experiencing a transformative period thanks to AI. From drilling and excavation to transportation, AI-powered systems leverage geospatial data to navigate intricate mining terrains. Robotics company OffWorld recently announced its plans to commence orders for its AI-powered, swarming robotic mining systems in 2024.
This line of autonomous mining robots is designed for surveying surface and underground environments, excavation, material collection, hauling, and material processing. The company’s swarming approach to robotic mining is said to improve productivity and efficiency, claiming that its robots can excavate more than one million tons of ore per year in a single mining operation.
Challenges and Future Prospects
While machine learning in mining offers many benefits, there are two big industry concerns: public perception and an equitable path forward.
Experts agree that the mining industry is unprepared for a future where machines replace the human workforce. According to the experts, society must recognize that mining is the foundation of sustainable development. The industry must also work to ensure that it produces benefits for the entire society.
These challenges present opportunities for innovative businesses capable of reimagining how mining can holistically evolve through the successful integration of machine learning. Efforts are also being made to ensure that mining better facilitates a more sustainable future by focusing on the extraction of minerals for electric vehicle batteries.
References and Further Reading
Chen, H., et al. (2023). Gis-Based Mineral Prospectivity Mapping Using Machine Learning Methods: A Case Study from Zhuonuo Ore District, Tibet. Ore Geology Reviews. doi.org/10.1016/j.oregeorev.2023.105627.
K-Mine. (2023). Digging Deeper with Data: Machine Learning in Ore Grade Estimation. [Online] K-Mine. Available at: https://k-mine.com/articles/digging-deeper-with-data-machine-learning-in-ore-grade-estimation/.
Picterra. (2023). How to Mitigate Risks in Mining Operations with Geospatial Intelligence. [Online] Picterra. Available at: https://picterra.ch/blog/how-to-mitigate-risks-in-mining-operations-with-geospatial-intelligence/.
Pell, R. (2023). AI-Powered Swarm Robots Aim to Disrupt Mining Industry. [Online] EE News. Available at: https://www.eenewseurope.com/en/ai-powered-swarm-robots-aim-to-disrupt-mining-industry/.
Sun, G., et al. (2022). Machine Learning Coupled with Mineral Geochemistry Reveals the Origin of Ore Deposits. Ore Geology Reviews. doi.org/10.1016/j.oregeorev.2022.104753.
Disclaimer: The views expressed here are those of the author expressed in their private capacity and do not necessarily represent the views of AZoM.com Limited T/A AZoNetwork the owner and operator of this website. This disclaimer forms part of the Terms and conditions of use of this website.