Predicting mining conditions is a significant part of opening most new mine operations. In geotechnically complex sites, underground, and in remote locations, predicting mining conditions is particularly challenging – and especially important.
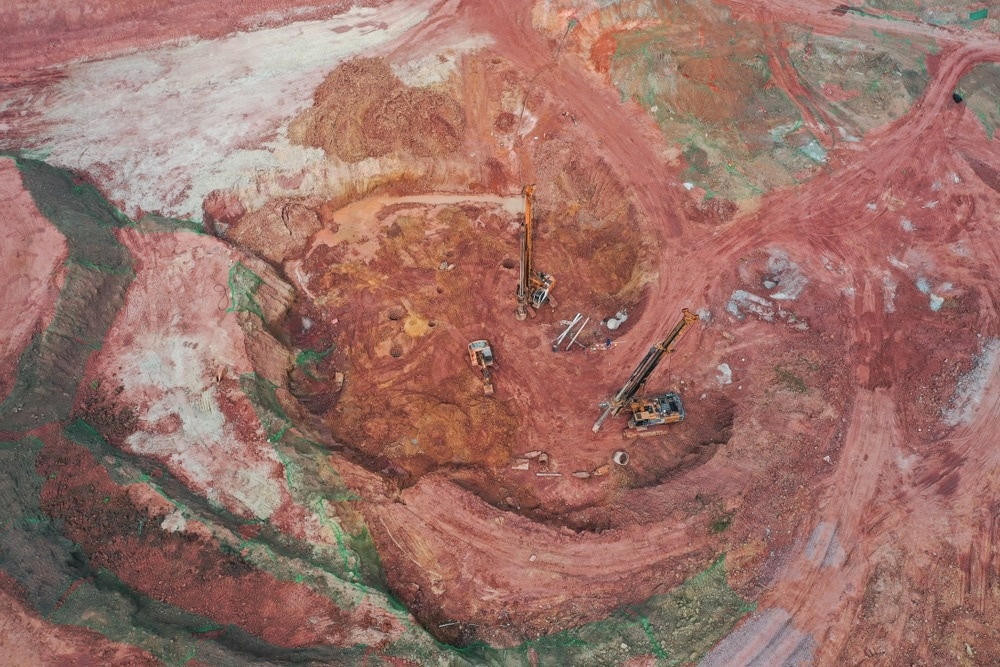
Image Credit: bqmeng/Shutterstock.com
Understanding Geotechnical Complexity
A significant cost involved in open pit mining is waste stripping. Waste stripping removes more material than is needed to expose the target ore or resource.
To avoid this, open pit mine operators try to make the pitches of the slopes at the side of the pit as steep as possible. This reduces the stripping ratio and by extension, the mine’s operational costs.
But steep slope angles can also increase the likelihood of the slope collapsing. Large-scale slope failure incurs significant clean-up costs, disrupts mine operations, damages mining equipment, and even causes a loss of mine reserves in some cases.
Geotechnical engineers must balance optimizing the slope angles for productivity and maintaining an acceptably low risk for significant slope failures.
Due to technological developments in mining machinery and structural engineering, open pit mines are now larger and deeper than ever. But this has also introduced more geotechnical complexity into planning operations.
The gamble that new mining operations represent is a bet by the mine’s financial backers. That bet is measured in purely financial terms, and so are the calculations about slope stability and general mining conditions that go into planning the new venture.
This means that geotechnical complexity needs to be translated into economic terms. Mining engineers and geologists have access to a wide-ranging toolbox of different predictive techniques and methods.
Artificial Neural Networks
There is already a wealth of data on strip mining operations worldwide, their development over time, and how different geotechnical complications impacted the long-term stability of their slopes.
A new method was recently introduced in a paper published in the journal Mining, which uses artificial neural networks (ANNs), a form of artificial intelligence (AI), combined with data modeling techniques to help predict mining conditions in geotechnically complex sites.
The team created a high-level causal model to account for different parameters affecting mining operations, such as hydrogeological conditions, meteorological conditions, and previous mine operations in the area.
They then trained an ANN on this historical data model alongside current production data. The ANN was capable of predicting future production based on the observable condition of the mine and then comparing its prediction with ongoing real data from the mine.
The team demonstrated that this approach was potentially effective and efficient by testing it on semi-synthetic data based on a real-world mine.
Gathering Data On Mining Conditions
Modern technology and methods – such as ANNs and other data-driven approaches – are beginning to predict conditions with unprecedented accuracy.
Professional geologists studying how the earth’s crust evolves – much like ANNs studying how mines have developed historically – can use prior knowledge to estimate how rocks and seams have formed underground.
For example, certain geological conditions favor the accumulation of certain types of ore – so geologists are already able to look at the most likely locations for target materials.
The surface will sometimes be eroded in such a way as to reveal the presence of target materials and likely extension of their deposits underground.
If not, geologists can conduct surveys with non-penetrating techniques such as seismic testing, magnetic analysis, or gravity testing.
If these steps reveal more ore or favorable conditions, the geologists will take core samples from the ground. Geologists analyze whole cores and cuttings from the drilling process to see if target materials are present.
Seismic Survey
Geologists can make relatively crude images of mining conditions below the surface using seismic reflection geophysics. This technique is particularly relevant for oil surveys, where concave-up features underground potentially hold liquid deposits.
Magnetic Survey
A magnetic survey can be employed to measure localized changes in the planet’s magnetic field. This can be caused by sedimentary rocks or mineral ore bodies underground. These materials have recognizable magnetic properties that make them relatively easy to identify and map in a magnetic survey.
Gravity Survey
Gravity surveys use a gravimeter to record minute changes in the localized gravitational field. Changes in the gravimeter’s acceleration are attributable to changes in the subsurface geological composition.
Physical Inspections
Physical inspections such as core test samples and small-scale exploratory digs contribute more verifiable data to predicting mining conditions.
This task is ongoing throughout the mine’s lifecycle, as more data enables more accurate predictions and, ultimately, a more significant windfall for investors.
Read more: Preventing Sinkholes in Mining: Recent Developments in the Industry
References and Further Reading
Elmouttie, M., J. Hodgkinson, and P. Dean (2021). Prediction of Mining Conditions in Geotechnically Complex Sites. Mining. doi.org/10.3390/mining1030018.
Jele, R., and M.J. Dunn (2019). Economic significance of geotechnical uncertainties in open pit mines. Proceedings of the First International Conference on Mining Geomechanical Risk. doi.org/10.36487/ACG_rep/1905_04_Jele.
Magnetic Survey - Mining Fundamentals. (2014) [Online] AZO Mining. Available at: https://www.azomining.com/Article.aspx?ArticleID=1255 (Accessed on 22 June 2022).
Disclaimer: The views expressed here are those of the author expressed in their private capacity and do not necessarily represent the views of AZoM.com Limited T/A AZoNetwork the owner and operator of this website. This disclaimer forms part of the Terms and conditions of use of this website.