Researchers in Kazakhstan recently developed a smart safety system for underground miner tracking. The machine learning-based technology uses Wi-Fi spot signals and inertial measurement unit (IMU) sensors to track miners’ whereabouts underground, and could drive up safety standards in mines worldwide.
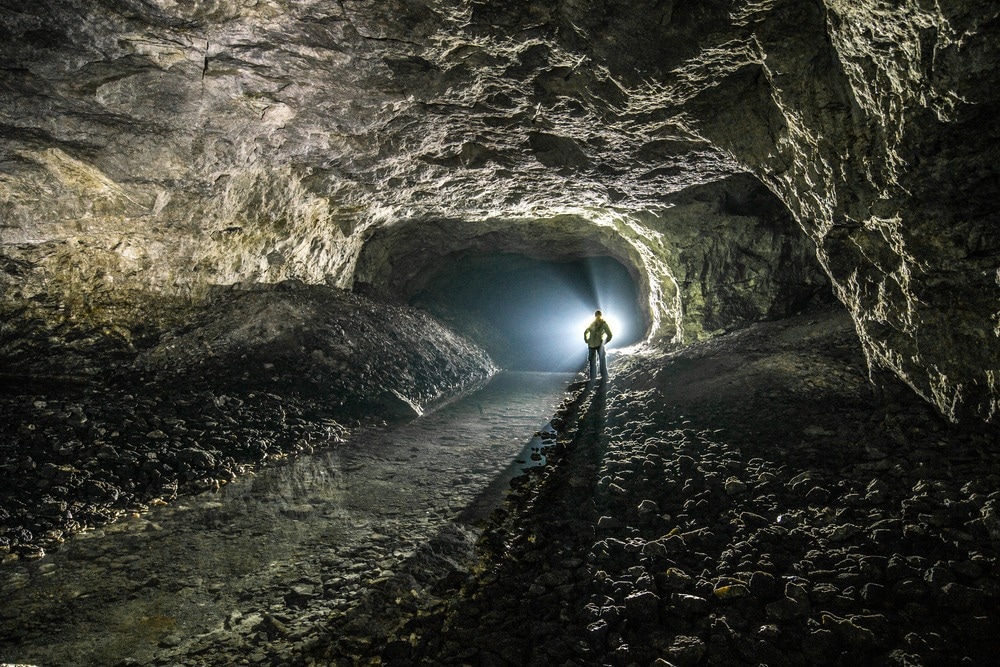
Image Credit: Marianna Ianovska/Shutterstock.com
Manual Underground Miner Tracking: Problems Beneath the Surface
Underground mining operations use manual tracking systems to monitor their miners’ locations. This job is usually carried out by the mine foreman who works with a dispatcher to draw up a precise list of everybody’s name and location in the mine.
If miners need to move to a different work area within the mine, they must contact the dispatcher using a tethered phone connection. The dispatcher then updates the location list accordingly.
Manual tracking systems have several drawbacks, with the US Department of Health & Human Services even warning against using them.
For example, the department says that if the work area that has been designated for a miner is too large, it will not be possible to pinpoint their location. The system is vulnerable to human error and has no fail-safe backup safety measures to detect or prevent failures in the safety system.
The US government passed the MINER Act in 2006. This requires coal mines to put electronic tracking systems in place to enable rescue operations to take place if necessary.
Electronic Underground Miner Tracking Systems
Electronic tracking systems are making their way into increasingly more mines every year. But currently available electronic methods such as GPS and Wi-Fi tracking still have some limitations.
Underground and open-cut mines present terrain restrictions for GPS and Wi-Fi sensing devices. Similarly, these systems struggle in disaster zones where the existing infrastructure has been destroyed.
Reader-based tracking can overcome these limitations. Using the same radio frequency identification (RFID) that prevents retail theft, mines can create ultra-low-cost electronic tracking systems over relatively small-scale operations.
Node-based electronic tracking technology creates a communications link between the radio and nodes. A chip analyzes the signal strength coming from a radio carried by the miner and determines the miner’s distance from a number of nodes around it to triangulate the miner’s location in three-dimensional space.
One proposed technology for miner tracking is known as inertial navigation or inertial guidance. Systems like these are carried by miners and passively measure accelerations, turns, and so on to calculate the miner’s distance from a fixed start point.
New Algorithm for Wi-Fi and IMU Method Could Be Used in Underground Mining Safety
Researchers at the Nazarbayev University’s Institute for Smart Systems and Artificial Intelligence in Kazakhstan recently developed a neural network algorithm designed to improve Wi-Fi-based localization and tracking systems.
The researchers say that their method, which combines data from Wi-Fi localization with IMU sensors data gathered in a handheld device, could improve mining safety by making a cost-effective smart tracking method more accurate, even with a poor Wi-Fi signal.
The algorithm was trained initially within the university building, which researchers used to simulate an underground mine.
By sending pairs of “workers” around the building, the team established a dataset with geospatial information from three floors using only information from Wi-Fi signals. They continued to “train” the algorithm that was generating a model of the building until it had an error distance of approximately 2.5 meters.
At this point, the researchers added data from IMU sensors to the algorithm’s feed.
IMU sensors will mean the tracking devices will still be able to localize themselves when the algorithm feed is offline by calculating the distance it has been displaced through IMU signals. This can give an overall estimate of the device’s position, which will become more accurate over time as the algorithm matures.
IMU sensors are already found in smartphones and pedometers. They are relatively inexpensive devices used to measure acceleration relative to the motion of the earth to calculate location.
The research is now focused on gathering more data to train the artificial neural network algorithm using machine learning. This process involves walking around the university building with handheld devices, feeding Wi-Fi access and IMU information back to the neural network.
The machine learning element will continue to refine the algorithm as more real-world data is fed into it. Researchers say this should result in a robust algorithm that can withstand the demands of localization in an underground mine.
While the neural network is still “growing,” the team is using fiducial markers that enable camera sensors to calculate their precise position relative to the marker. This provides real-world feedback for the algorithm to develop itself with machine learning.
In the future, the team plans to incorporate 5G connectivity into the system. This will increase the capacity of networks based on their system because 5G networks have a much higher density than Wi-Fi.
References and Further Reading
Cholteeva, Y. (2021). Out of thin air: tracking underground miners in poor connection areas. [Online] Mining Technology. Available at: https://www.mining-technology.com/ (Accessed on 20 May 2022).
Electronic Tracking Systems Performance. [Online] CDC. Available at: https://www.cdc.gov/niosh/mining/content/emergencymanagementandresponse/commtracking/advcommtrackingtutorial3.html (Accessed on 20 May 2022).
Khassanov, Y, et al. (2021). Finer-level Sequential WiFi-based Indoor Localization. 2021 IEEE/SICE International Symposium on System Integration. doi.org/10.1109/IEEECONF49454.2021.9382623.
Nurpeiissov, M., et al. (2022). End-to-End Sequential Indoor Localization Using Smartphone Inertial Sensors and WiFi. 2022 IEEE/SICE International Symposium on System Integration. doi.org/10.1109/SII52469.2022.9708854.
Disclaimer: The views expressed here are those of the author expressed in their private capacity and do not necessarily represent the views of AZoM.com Limited T/A AZoNetwork the owner and operator of this website. This disclaimer forms part of the Terms and conditions of use of this website.