Slope stability is essential in mining operations since slope failure endangers safety and productivity. The complexity of conventional geotechnical methods makes slope failure prediction challenging. Artificial intelligence (AI) has helped mining companies forecast slope failures quickly and efficiently through detailed analysis.
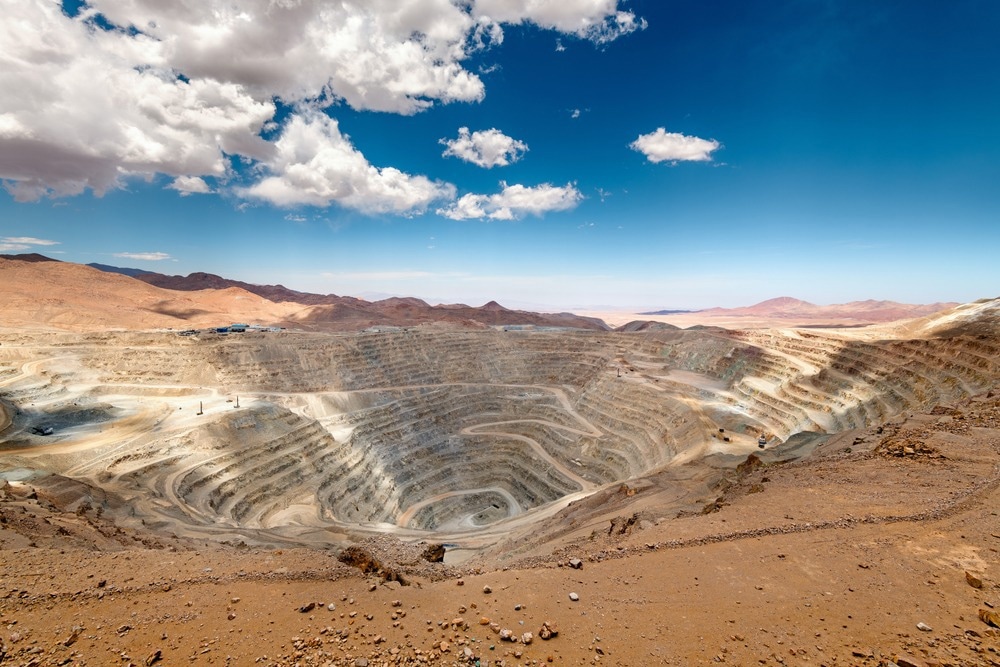
Image Credit: Jose Luis Stephens/Shutterstock.com
Slope Failure in Mining
Due to the development of more advanced mining techniques and the growing demand for mineral resources, most mines are constructed to extract more minerals from steeper or deeper areas.
The steeper slope angle makes these mines more vulnerable to slope failure. It can cause injury to workers, damage to mine equipment, and halt production, negatively influencing mining productivity.
How Can Slope Failures Be Prevented?
Slope failures are prevalent in mines, becoming increasingly difficult to avoid as the excavation gets more profound and challenging to control.
Understanding rock mass characteristics, structural geology, the impact of groundwater pressure, and other local external forces in detail is necessary for reducing the danger of slope failure.
Excavation walls are kept as steep as possible to maximize operating efficiency, which can cause slope failures. However, most slop failures are caused by shear and fracture on existing defects.
The best strategy to prevent such failures is to maintain the factor of safety (FOS) within a reasonable range (>1). In situations where the factor of safety is exceptionally low, extra consideration should be paid to prevent possible slope failure.
Slope Monitoring in Mining
The behavior of the rock structure during mining must be monitored to assess whether rock slopes pose a danger to workers' safety.
The ability to send warnings before large-scale rock movement or a possible failure is essential for a successful monitoring system.
Traditional Geotechnical Instruments for Slope Failure
Traditionally, slopes have been monitored using geotechnical instruments such as piezometers, borehole extensometers, crack meters, and stress meters.
Even though there has been significant development in geotechnical monitoring instrumentation, such as remote-sensing technologies, these processes have limitations that make predicting slope failure exceedingly difficult.
Role of Artificial Intelligence in Determining Slope Failure
Even though geotechnical techniques have successfully reduced slope failure incidents, they are complicated and involve various elements, including geological activity, ground conditions, and human behavior, which are dynamic and constantly changing. Hence, it is difficult to measure them consistently.
However, the introduction of AI in mining has tremendously benefited engineers in predicting potential slope failure through in-depth analysis.
Artificial intelligence can automatically detect factors influencing slope failures, such as bedding planes, spaces, shear zones, and fractures on the bench face. AI can spot cracks that emerge before a slope failure and toe deformations that indicate rock movement on the Earth.
By comparing daily data, AI predicts failures in the existing slope and other geologies. The AI can keep track of the data to determine not only the best slope to avoid failure but also the events that may build up to and trigger a failure in the future.
Current Research in Using AI for Slope Failures
The research that used AI to determine safety parameters in mines has shown to be successful and efficient in correctly predicting slope failures. Furthermore, AI models can process massive amounts of data simultaneously and provide predictions faster than traditional stability analysis methods.
Artificial neural network (ANN) model
Kothari and Momayez's study evaluated the predictive capabilities of the artificial neural network (ANN) and inverse velocity (IV) models to anticipate the slope collapse of an open-cast mine. They created a double-layered feed-forward ANN utilizing 22 datasets gathered using radar equipment.
The investigation showed that ANN prediction was more accurate than the inverse velocity model by 86%. Total slope failure forecasts showed that 82% of the slopes were safe, while 18% showed that the slopes were dangerous. Moreover, the ANN model provided unsafe predictions five minutes before the actual failure, indicating that the AI model is much safer than the IV model.
Back-propagation neural network (BPNN) model
In a study by Ferentinou and Fakir, a Back-propagation Neural Network (BPNN), an AI algorithm, model was created using 141 databases collected from surface mine cases worldwide and 18 input variables, including hydraulic profile, rock mass characteristics, environmental factors, geological features, blast design, slope dimensions, and the occurrence of previous instability.
This study investigated all slopes to anticipate failure to establish slope stability indices. The results suggested using BPNN as a reliable method to predict slope failure in feasibility studies, with a mean square error value of 0.0001 converging at 98%.
Recent Developments: IDS GeoRadar
IDS GeoRadar has introduced Ai.DA, a new solution for geotechnical professionals that utilizes artificial intelligence to monitor slope stability.
Ai.DA anticipates possible slope failure by analyzing the compatibility of the detected rock movement trend with established slope instabilities models and behaviors, enabling experts to optimize operations and make intelligent judgments.
Ai.DA assists users in deciphering large amounts of data intelligently. It is fully incorporated with Guardian, IDS GeoRadar's user-friendly and powerful program for managing slope stability issues in mining operations.
Angela Patera, Guardian Product Owner at IDS GeoRadar, said, "With Ai.DA, we are pioneering the use of artificial intelligence in slope stability monitoring, and we commit to providing customers with state-of-the-art technology solutions to support radar data interpretation."
Future Outlooks of Slope Failure Monitoring
Technology advancements have improved slope monitoring methods and enabled the evaluation of slope stability over time to provide insight into slope dynamics. In operational open-pit mines, it is crucial to accurately predict slope failure to avoid it and protect machinery and workers from harm. It is essential to evaluate the slope stability-affecting factors while determining a slope's stability.
Numerous researches have demonstrated the ability of AI models to anticipate mining slope failure quickly and accurately. However, it is necessary to compare multiple AI models' outputs to identify the best accurate model for slope stability forecasting.
Read more: Using Artificial Intelligence for Mineral Processing and Exploration
References and Further Reading
Bui, X. N., Nguyen, H., Choi, Y., Nguyen-Thoi, T., Zhou, J., & Dou, J. (2020). Prediction of slope failure in open-pit mines using a novel hybrid artificial intelligence model based on decision tree and evolution algorithm. Scientific reports, 10(1), 1-17. https://doi.org/10.1038/s41598-020-66904-y
Ferentinou, M., & Fakir, M. (2018). Integrating rock engineering systems device and artificial neural networks to predict stability conditions in an open pit. Engineering Geology, 246, 293-309. https://doi.org/10.1016/j.enggeo.2018.10.010
Gleeson, D. (2022). IDS GeoRadar Adds Artificial Intelligence Layer to Slope Stability Monitoring Process. [Online] International Mining. Available at: https://im-mining.com/2022/04/27/ids-georadar-adds-artificial-intelligence-layer-to-slope-stability-monitoring-process/ (Accessed on 23 June, 2022)
Kolapo, P., Oniyide, G. O., Said, K. O., Lawal, A. I., Onifade, M., & Munemo, P. (2022). An Overview of Slope Failure in Mining Operations. Mining, 2(2), 350-384. https://doi.org/10.3390/mining2020019
Kothari, U. C., & Momayez, M. (2018). Machine learning: A novel approach to predicting slope instabilities. International Journal of Geophysics, https://doi.org/10.1155/2018/4861254
Disclaimer: The views expressed here are those of the author expressed in their private capacity and do not necessarily represent the views of AZoM.com Limited T/A AZoNetwork the owner and operator of this website. This disclaimer forms part of the Terms and conditions of use of this website.