The age-old practice of ore sorting is getting a high-tech makeover thanks to advancements in sensor technologies. As sensors enable automated, real-time sensing of ore characteristics, they hold the potential to transform ore sorting into a highly optimized process that maximizes sustainability and efficiency.
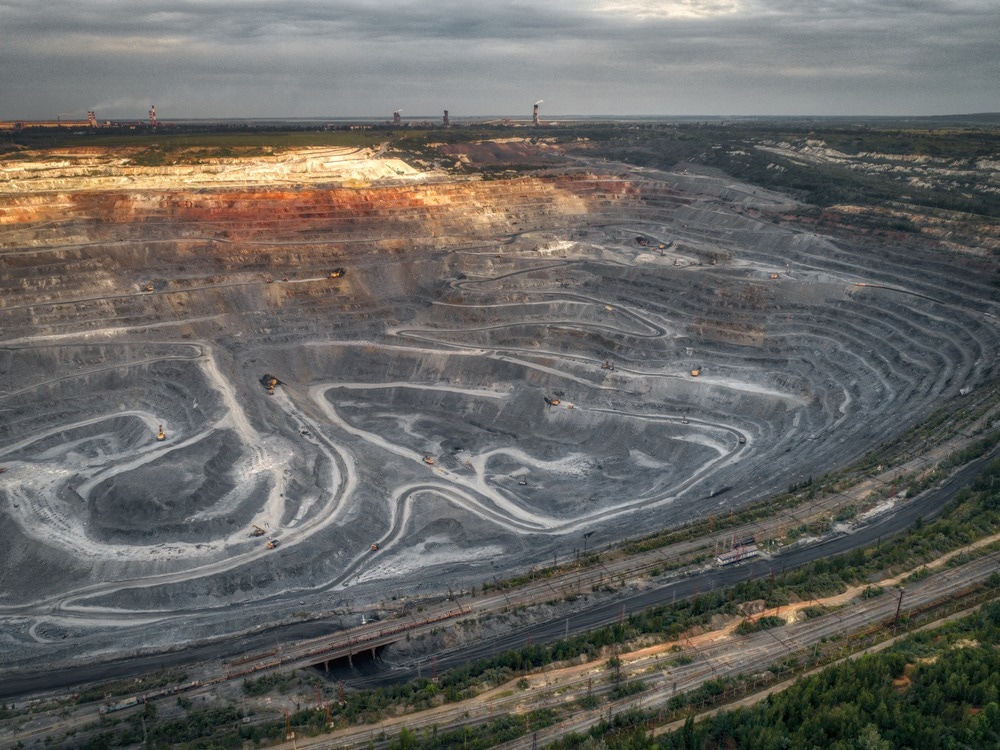
Image Credit: Nordroden/Shutterstock.com
The Significance of Ore Sorting
Ore sorting is the separation of mineral resources based on economic value, separating rock containing valuable commodities from low-grade waste material. This process allows mining companies to extract the most value by prioritizing high-grade ore for energy-intensive downstream processing while diverting gangue to waste piles early on.
Traditionally, workers manually inspected rocks on a conveyor belt and picked out valuable ore from waste gangue minerals. However, manual sorting is imprecise, inefficient, costly, and limited by the accuracy of human judgment.
The introduction of advanced sensors has enabled automated sorting with far greater throughput, speed, and precision. They analyze individual particles or bulk streams in real-time, guiding automated ejectors to selectively sort and divert waste rocks. This minimizes the processing of barren material, enhancing the recovery of valuable minerals from low-grade or complex ores. It also allows the reclamation of residual materials from mine waste and tailings, unlocking value from previously discarded material.
There are two main sensor-based ore sorting approaches:
- Bulk sorting: Sensors analyze characteristics of the bulk stream on the conveyor, such as average atomic density. If the analyzed section meets threshold criteria, the entire bulk is diverted. This allows high-capacity sorting of over 10 tons per trigger.
- Particle sorting: Each particle (larger than 30mm) is individually sensed and sorted on a conveyor belt, allowing valuable particles to pass while ejectors remove waste rocks. This enables precise sorting at capacities over 150 t/h per meter.
Advancements in Sensor Technologies
Several sensing technologies are driving the improvements in precision and automation of modern ore sorting:
X-Ray transmission sensors
X-Ray transmission (XRT) sensors differentiate ores based on material density and absorption characteristics when penetrated by X-Rays. This enables the automated sorting of indistinguishable minerals through optical methods alone. In addition, applying XRT scanning early in the process flowsheet maximizes separation efficiency while protecting downstream crushing and grinding equipment from barren rock ingress.
Advanced XRT sensors such as Steinert XSS T EVO 5.0 feature dual energy X-Ray transmission, enabling them to penetrate ores up to 100 mm and distinguish between pure copper and copper alloys like brass based on subtle variations in density and energy absorption. This enables mining firms to separate high-purity copper from ore once considered low-grade and mixed.
Near-infrared sensors
Near-infrared (NIR) sensors analyze different minerals' unique spectral reflection signatures to identify and differentiate between ore types. Its non-destructive nature delivers a rapid assessment of mineral identity without any material loss. This high-speed sensing method is a major driver of automated ore sorting.
NIR scanning sensors are used to sort diamonds, separating diamond-bearing kimberlite ore from barren rock based on distinct spectral signatures. This provides an alternative to traditional ore concentration methods, which incur substantial diamond losses during processing.
RWTH Aachen University implemented NIR sensors for borate mines, which increased borate grade from 38% to 45.45% and achieved an 85.40% yield, improving flotation outcomes.
Hyperspectral imaging
Hyperspectral imaging combines high-resolution spatial imaging with detailed spectroscopic data across narrow wavelength bands, generating unique spectral signatures for each pixel. It provides extensive material composition data and is particularly valuable in ore sorting, enabling precise mineral identification.
When deployed near mining sites or on conveyors, hyperspectral imaging sensors enhance ore characterization, sorting decisions, and automated ore diversion control, surpassing traditional bulk material spectroscopy techniques.
Sustainability Benefits of Sensor-Based Ore Sorting
Reduced environmental impact
Implementing sensors for ore sorting enables a more targeted, efficient mining process that greatly reduces environmental impacts. Sensors perform real-time rock property analysis, distinguishing high-grade ore from waste rock. This minimizes the ore mining and processing required to extract the same target mineral quantities.
This precision results in a lower volume of material sent to waste piles, leading to an overall reduction in waste generation. With less waste generation, there is reduced demand for land reclamation and mitigation of waste pile impacts like acid rock drainage.
The early sorting of barren rock improves resource efficiency and lowers greenhouse gas emissions by eliminating the need for unnecessary mechanical and chemical processing.
Lower energy consumption
Sensor-based sorting technology enhances operational efficiency by optimizing energy, water, and reagent usage per ton of product, reducing resource consumption. This is vital in increasing competition for resources from neighboring communities and agriculture, which is pivotal in securing social acceptance for mining operations.
Early identification and removal of uneconomical ore cuts unnecessary, energy-intensive crushing and grinding, leading to an estimated 50% reduction in energy consumption, thereby improving mining sustainability and efficiency.
Efficiency Gains: Higher Recovery Rates and Cost-Savings
Automated sensor-based ore sorting substantially enhances productivity and efficiency, consistently recovering 5-20% more desired minerals than manual sorting. Selectively removing waste gangue lightens the processing plant's load, allowing for a higher throughput of valuable ore and increased overall production rates.
For instance, in South Africa, P2E Consulting replaced an existing drum-dense media separation (DMS) plant at Eastern Chrome mines with a TOMRA X-Ray Transmission sorter. This technology efficiently upgraded under-value material, enhancing its grade from 20% to 28% to a saleable product with a minimum grade of 38% Cr2O3. The outcomes were noteworthy, demonstrating chromium grades surpassing 40% Cr2O3 and achieving mass recoveries ranging from 25% to 30% from the separated waste. Consequently, Chrome-in-tails were minimized to as low as 12%.
Notably, this approach eliminates water usage and the need for expensive reagents, resulting in a small lumpy product produced at approximately 50% of the cost compared to a drum-dense media separation (DMS) plant, showcasing the cost-saving potential of sensor-based sorting.
Sensor sorting technology also replaces labor-intensive manual processes, offering several cost-saving advantages. It reduces energy-intensive grinding, cuts transportation and waste management costs, and eliminates the need for expensive reagents. Even a modest 30% removal of gangue from the feed results in substantial energy savings during grinding.
Mining waste repurposed as construction material, facilitated by sensor-based sorting, enhances sustainability and boosts revenue, as demonstrated by China's Fankou mine, which gained $9.22 million in annual revenue through such practices. The integration of HPY's technology, featuring dual-energy X-Ray technology sensors and high-speed air jets, enabled zero-waste operations and generated an extra $9 million in annual revenue from 1.4 million tons of ore production.
Challenges and Considerations
While sensor-based ore sorting offers numerous advantages, there are significant challenges tied to its implementation, primarily focused on the initial investment and data processing:
Initial investment: Sensor-based ore sorting requires substantial investment for equipment, infrastructure, and possibly plant modifications, depending on the mining operation's size and needs. This necessitates careful planning and design efficient implementation.
A significant part of the initial investment is allocated to workforce training, including specialized training for equipment operators, maintenance personnel, and data analysts to ensure the proper operation, maintenance, and effective data interpretation.
While this initial investment in training and equipment can be substantial, it yields long-term benefits through increased efficiency and reduced operating expenses.
Data processing: Managing the substantial data generated by sensor-based ore sorting, which includes ore characteristics like size, shape, density, and composition, poses a significant challenge, particularly in large-scale mining. Effective data analysis is vital for real-time sorting decisions, necessitating advanced techniques and tailored software.
To address this, companies must invest in tailored data analysis tools, seamlessly integrate sensor-based ore sorting data with existing systems, and implement robust measures to protect sensitive information, ensuring confidentiality and integrity.
Concluding Remarks
While new challenges exist, the efficiency gains and sustainability benefits provided by sensor-based ore sorting make it a compelling technique worthy of the investment and planning required to implement it successfully. Mine operators who leverage high-fidelity sensor data will be best positioned to maximize resource efficiency and productivity. With thorough analysis and foresight, sensor-based ore sorting can deliver transformative value.
Continue Reading: The Environmental and Economic Benefits of Ore Sorting
References and Further Reading
Luo, X., He, K., Zhang, Y., He, P., & Zhang, Y. (2022). A review of intelligent ore sorting technology and equipment development. International Journal of Minerals, Metallurgy and Materials, 29(9), 1647-1655. https://doi.org/10.1007/s12613-022-2477-5
Steinert Global. (2023). Efficiently optimise ore sorting - Use ore sorting equipment to concentrate ores and cut process costs. [Online]. Available at: https://steinertglobal.com/mining/ore-sorting/
Peukert, D., Xu, C., & Dowd, P. (2022). A Review of Sensor-Based Sorting in Mineral Processing: The Potential Benefits of Sensor Fusion. Minerals, 12(11), 1364. https://doi.org/10.3390/min12111364
Metso. (2023). Ore sorting: the road to optimizing your operation. [Online]. Available at: https://www.metso.com/insights/blog/mining-and-metals/ore-sorting-the-road-to-optimizing-your-operation/
Sharma, A. (2023). How can sensor-based ore sorting turn waste into wealth? [Online]. Available at: https://www.mining-technology.com/features/sensor-based-sorting-in-mining-companies/?cf-view
Shatwell, D. G., Murray, V., & Barton, A. (2023). Real-time ore sorting using color and texture analysis. International Journal of Mining Science and Technology. https://doi.org/10.1016/j.ijmst.2023.03.004
Thomson, E. (2022). Opportunities for Improvement: An Introduction to Ore Sorting. [Online]. Available at: https://www.thermofisher.com/blog/mining/opportunities-for-improvement-an-introduction-to-ore-sorting/
Tomra Mining. (2023). Achieving a circular economy with TOMRA's sensor-based ore sorting technology. [Online]. Available at: https://www.tomra.com/en/mining/media-center/news/2020/2020-circular-economy
Disclaimer: The views expressed here are those of the author expressed in their private capacity and do not necessarily represent the views of AZoM.com Limited T/A AZoNetwork the owner and operator of this website. This disclaimer forms part of the Terms and conditions of use of this website.