In a recent article in Sustainability, researchers developed a predictive model for short-term methane concentrations at the longwall outlet using a Multi-Layer Perceptron (MLP) neural network.
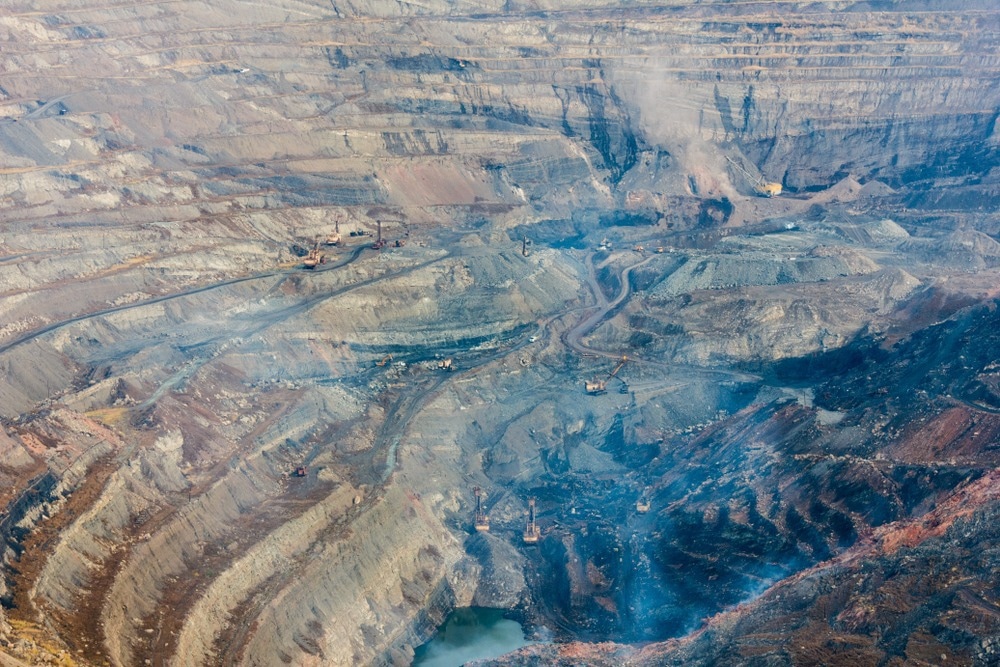
Image Credit: Alexander_Z/Shutterstock.com
This model leverages data from an automatic gas measurement system, aiming to enhance safety measures in mining operations by providing timely forecasts of methane levels. The study emphasizes the importance of accurate and rapid predictions to facilitate immediate responses to hazardous situations, improving overall mine safety.
Background
The underground coal mining industry faces significant challenges related to methane emissions, which pose serious safety risks, including the potential for explosions and health hazards for miners.
Methane, a highly flammable gas, can accumulate in confined spaces, making real-time monitoring and management essential for safe mining operations. Traditional methods of monitoring methane levels often lack the responsiveness and accuracy required to address these risks effectively.
As mining operations become more complex and deeper, the need for advanced predictive tools to provide timely methane concentration forecasts is increasingly critical. This study aims to fill the gap in existing research by employing a Multi-Layer Perceptron (MLP) neural network to predict short-term methane levels based on data from automatic gas measurement systems.
The Current Study
The methodology employed in this study involved developing a Multi-Layer Perceptron (MLP) neural network to predict methane concentrations at the longwall outlet in underground coal mining. The process began with acquiring real-time data from the mine's automatic gas measurement system, which provided critical information on methane levels. This dataset was then prepared by averaging the measurement values over one-minute intervals to ensure consistency and reliability.
The dataset was divided into two subsets to enhance the model's performance: 70% was allocated for training the neural network, while the remaining 30% was reserved for testing and validation. This division aimed to prevent overfitting, ensuring the model could generalize well to unseen data. The MLP architecture was designed with an input layer corresponding to the number of features from the gas measurement data, a hidden layer with 26 neurons, and a single output neuron representing the predicted methane concentration.
The training process involved adjusting the network's weights using back-propagation and minimizing the mean squared error as the loss function. The model's effectiveness was evaluated based on its ability to predict methane concentrations across various time intervals. The model's predictions were validated by comparing the forecasted values against actual measurements, focusing on maintaining a Mean Absolute Percentage Error below 18%. This rigorous methodology aimed to provide a robust tool for enhancing safety in underground mining operations by enabling timely responses to hazardous methane levels.
Results and Discussion
The study's results demonstrate the effectiveness of the MLP neural network in predicting methane concentrations at the longwall outlet. The highest accuracy was observed in the 5-minute forecasts, where the Mean Absolute Percentage Error (MAPE) remained below 10%, indicating a strong correlation between predicted and actual methane levels.
As the forecasting horizon increased, the accuracy of the predictions decreased, with the 60-minute forecasts exhibiting the most significant errors yet still maintaining a MAPE below 18%. The Pearson correlation coefficients across all time intervals consistently indicated a strong relationship between the predicted and observed values, reinforcing the model's reliability.
Additionally, the Index of Agreement (IA) scores further validated the model's performance, showcasing its capability to provide actionable insights for real-time methane monitoring. The results highlight the MLP network's adaptability and robustness in dynamic mining environments, emphasizing its potential as a practical tool for enhancing safety management. Overall, the findings suggest that the developed methodology can significantly improve the forecasting of methane concentrations, thereby contributing to safer mining operations and effective risk mitigation strategies.
Conclusion
In conclusion, the research presented in the article offers a promising approach to predicting methane concentrations in underground coal mining through the use of Multi-Layer Perceptron neural networks.
The developed model harnesses data from automatic gas measurement systems to provide rapid and accurate forecasts for ensuring miner safety.
The study highlights the importance of real-time data in enhancing the adaptability and effectiveness of predictive models. The findings suggest that implementing such advanced forecasting techniques can significantly reduce the risks associated with methane accumulation in mines.
As the mining industry evolves, adopting innovative solutions like the MLP model will be essential in addressing safety challenges and promoting sustainable practices. The authors advocate for further research to refine these methodologies and explore their application in various mining contexts, ultimately contributing to a safer working environment for miners.
Source:
Tutak M., Krenicky T., et al. (2024). Predicting Methane Concentrations in Underground Coal Mining Using a Multi-Layer Perceptron Neural Network Based on Mine Gas Monitoring Data. Sustainability 16(19):8388. DOI: 10.3390/su16198388, https://www.mdpi.com/2071-1050/16/19/8388