The mining industry relies heavily on large, complex machinery for extracting and processing resources. Keeping this expensive equipment in optimal working condition is critical for ensuring efficient, uninterrupted operations, reducing downtime, improving safety, and maximizing productivity. Implementing proactive, preventive maintenance strategies centered around prediction and prevention is key to mining success in today's data-driven landscape.
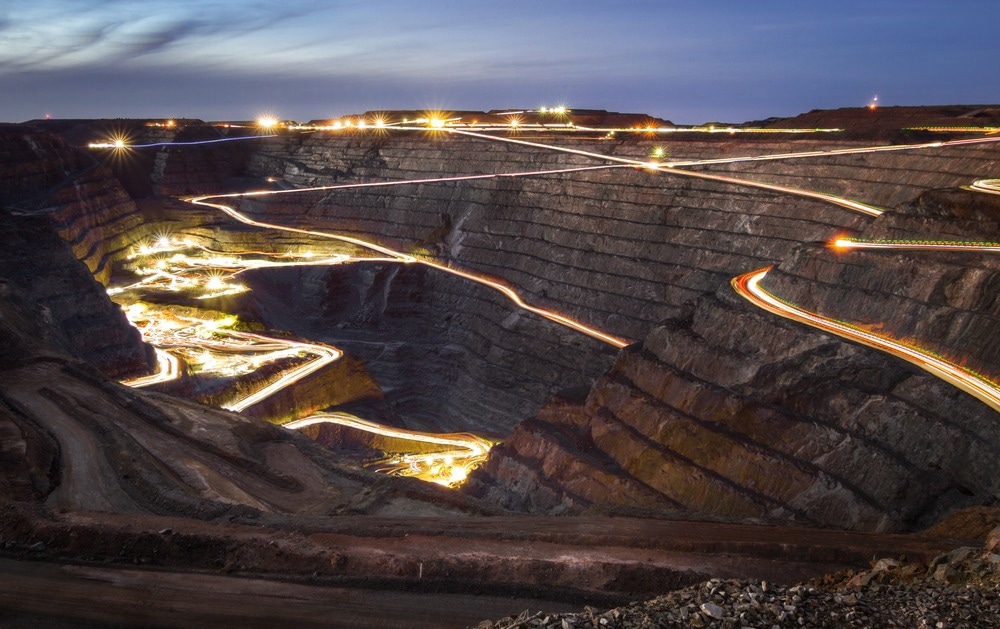
Image Credit: Christoph Schaarschmidt/Shutterstock.com
Mining has a long history, dating back to ancient Egyptian civilization. Today, millions work in the global mining industry, with significant investments in equipment production. The industry is increasingly mechanized and automated to meet production targets, making equipment more complex and costly.
Effective maintenance management ensures mining equipment consistently operates at peak efficiency with minimum disruptions for a long time.
Preventive Maintenance
Implementing a robust preventive maintenance program based on regular, scheduled service and inspections is a fundamental strategy mining companies must embrace. Instead of simply reacting to problems as they occur and forcing downtime, preventive maintenance takes a proactive approach to identify and mitigate issues before a breakdown.
Scheduled maintenance activities like component replacement or lubrication at predetermined intervals prevent deterioration and ensure equipment reliability. Adhering to maintenance schedules recommended by OEMs based on factors like operating hours, mileage, or calendar time maximizes availability while minimizing repair costs over the lifetime.
Predictive maintenance augments scheduled upkeep by leveraging technologies to anticipate problems. Sensor data can be analyzed for early warning signs of failure, like high vibration or temperature, allowing maintenance to be planned before a breakdown.
Preventive maintenance is proven to offer tremendous advantages. Studies indicate it can reduce downtime by 30-50%, lower maintenance costs by 5-10%, and decrease equipment failures by 70-75%. Making these changes can lead to major productivity and profit gains.
Condition Monitoring
While preventive maintenance is time-based, condition-based monitoring is measurement-based. It leverages sensors and analytics to continuously assess the actual operating condition of equipment based on parameters like vibration, temperature, noise, pressure, and lubricant quality.
Establishing baseline thresholds for these metrics allows deviations to be detected early, and emerging faults can be identified before performance is impacted. This enables mining companies to schedule maintenance judiciously before failure, not after.
For example, a rise in vibration levels may indicate misalignment or loose parts, and elevated temperature can signify blocked cooling systems or overloading. At the same time, particle counters detect bearing wear through increased contaminants in lubricant samples.
The advent of low-cost sensors, internet of things connectivity, data storage and advanced analytics provides powerful predictive capabilities to boost equipment reliability. Around-the-clock monitoring also optimizes maintenance labor allocation and reduces inspection costs.
Equipment Reliability
Maintaining reliable machinery is crucial in the evolving landscape of the mining industry. Well-maintained equipment experiences less unexpected downtime, performs optimally per engineered capacity, and enjoys longer service life between overhauls. In addition, enhanced equipment reliability also translates directly into improved safety, as unreliable assets are more prone to potentially dangerous operational incidents.
Investments in reliability give substantial returns by minimizing disruptive failures and driving productivity gains – average savings from improvements in reliability are estimated to be over 5% of revenues.
Training and Skill Development
An experienced, skilled workforce is indispensable for the complex task of heavy mining equipment maintenance. Thorough training and development prepare personnel to handle various maintenance functions efficiently – inspections, diagnostics, repair procedures, preventive scheduling, data analysis, and more.
With mining equipment becoming increasingly sophisticated, training must keep pace with technological advancements to build relevant expertise. Simulator training, immersive virtual reality environments, and digital learning platforms enable better skill development despite remote mining sites.
Investment into skills also improves motivation, morale and employee retention. Collectively harnessing individual competencies and experiences through teams facilitates rapid diagnosis and restoration of equipment functionality through quality repairs. These capabilities culminate in the organizational advantage of reliable equipment and lower costs.
Spare Parts Inventory Management
Since equipment breakdowns require immediate repairs, spare parts availability is critical to minimize downtimes in mining. However, finding the right inventory balance is difficult – stockouts lead to outages, but excess stock ties up capital.
By assessing part criticality, lead times, equipment maintenance schedules and other variables, optimized stock levels can be defined to avoid shortages while reducing holding costs.
Categorizing spare parts into groups and levels with varied management approaches also brings efficiencies – higher stocks for vital or long-lead items, lower inventories for standard parts, and pooling for expensive spares.
With optimized inventory, maintenance teams gain flexibility in scheduling repairs, money is freed up for other investments, and downtime costs are contained through part availability.
Asset Management Software
Asset management softwares, such as FTMaintenance and AMT, offer robust preventive maintenance planning and scheduling features, enabling organizations to track routine equipment inspections, lubrication of moving parts, and regular cleanings.
A digital library of maintenance documentation provides on-site technicians with easy access to warranty information, equipment manuals, and other critical maintenance documents. Real-time dashboards provide actionable insights into asset health and facilitate tracking maintenance key performance indicators (KPIs).
Collectively, these capabilities optimize labor productivity, reduce human errors, offer data-driven decision support, and enhance the overall efficiency, reliability, and cost-effectiveness of maintenance operations.
Environmental Considerations
Mining operations are widely recognized for their adverse environmental effects, including habitat destruction, water and soil pollution, air emissions, and resource depletion. However, adopting appropriate maintenance practices can be a crucial countermeasure, mitigating these detrimental impacts and advancing environmentally conscious mining activities.
Effective maintenance practices minimize resource wastage by keeping mining equipment in prime condition, reducing the need for replacements and conserving metals and minerals. They enhance energy efficiency, as well-maintained machinery consumes less energy, reducing the ecological footprint.
Addressing engine and exhaust systems in maintenance reduces harmful emissions and particulate matter, improving air quality at mining sites. Additionally, eco-friendly maintenance extends to tailings management, safeguarding against potential leaks or breaches that could contaminate soil and water.
Case Study: Rio Tinto's Cost-Saving Predictive Maintenance Approach
Rio Tinto operates some of the world's largest fleets of autonomous mining haul trucks. These massive vehicles, transporting iron ore 24/7 under extreme loads, are critical revenue generators. On average, each day a truck is out of service costs the company $2 million in lost revenue, and the expense can double when towing is needed to remove the truck from the pit. To address this challenge, Rio Tinto turned to IoT-driven predictive maintenance.
The company installed 92 sensors on its fleet of 900 large dump trucks, generating a staggering 4.9 terabytes of data daily by monitoring engine, drivetrain, and wheel conditions. The computers process this data in near-real time, employing rule-based algorithms and deep learning to identify, for instance, a 60 percent likelihood of a part failing within three weeks. An alert is then generated, enabling timely part ordering and vehicle servicing scheduling.
This approach has significantly reduced unplanned downtime, resulting in substantial cost savings.
Concluding Remarks
Modern mining demands intelligent maintenance centered on prevention and prediction to keep pace with increasingly complex equipment. Investments in skilled personnel, software tools, sensors, analytics, and inventory optimization allow mining companies to achieve new heights of safety, sustainability and profitability through equipment reliability. By embracing proactive maintenance, miners can realize substantial gains in productivity and efficiency.
Read More: Using Digital Twins for 3D Visualization of Deep-Sea Mining Operations
References and Further Reading
Benjamin Byrne & Chris Engdahl. (2021). Mining 4.0: How innovation is shaping mines of the future. [Online]. Available at: https://miningdigital.com/smart-mining/mining-40-how-innovation-shaping-mines-future
Dhillon, B. S. (2008). Mining Equipment Reliability, Maintainability, and Safety. Springer London. https://link.springer.com/book/10.1007/978-1-84800-288-3
FasTrak SoftWorks. (2023). Mining Asset Management Software. [Online]. Available at: https://ftmaintenance.com/industries/mining-and-natural-resources/
Finning International. (2023). Maximize the Productivity of Your Mining Operation With the Right Equipment Strategy. [Online]. Available at: https://www.finning.com/en_CA/company/news-events/product-customer-stories/maximize-the-productivity-of-your-mining-operation-with-the-right-equipment-strategy.html
Kranz, M. (2017). Go Big on IoT Value with Preventive Maintenance. [Online]. Available at: https://www.iotforall.com/go-big-iot-value-preventive-maintenance
Massonini Ngoma, R. G. T., Abraham Mahanga Tsoni, C. G., Meng, X., & Bashiru Danwana, S. (2023). The Impact of the Mining Equipment, Technological Trends, and Natural Resource Demand on Climate Change in Congo. Sustainability, 15(2), 1691. https://doi.org/10.3390/su15021691
MiningTechnology. (2023). AMT Asset Management Tool [Online]. Available at: https://www.mining-technology.com/products/amt-asset-management-tool/
Optimal. (2023). A Spares or Inventory Management Guide for Mining Operations. [Online]. Available at: https://www.linkedin.com/pulse/spares-inventory-management-guide-mining-operations-optimalworld/
Pardede, S. R., & Vanany, I. (2021). Analysis and Control for Heavy Equipment Spare Parts Inventory in the Nickel Mining Industry. IPTEK Journal of Proceedings Series, (6), 478-483. https://iptek.its.ac.id/index.php/jps/article/view/11146
Tomlingson, P. D. (2010). Equipment management: Key to equipment reliability and productivity in mining. SME. https://nibmehub.com/opac-service/pdf/read/Equipment%20Management%20_%20key%20to%20equipment%20reliability%20and%20productivity%20in%20mining.pdf
ToolSense. (2023). How to Improve Mining Equipment Maintenance and Safety. [Online]. Available at: https://toolsense.io/maintenance/how-to-improve-mining-equipment-maintenance-and-safety/
Tyrrell, P. (2023). Comprehensive Guide to Mining Equipment Maintenance. [Online]. Available at: https://www.fieldinsight.com/blog/comprehensive-guide-to-mining-equipment-maintenance/
Disclaimer: The views expressed here are those of the author expressed in their private capacity and do not necessarily represent the views of AZoM.com Limited T/A AZoNetwork the owner and operator of this website. This disclaimer forms part of the Terms and conditions of use of this website.